Update TextBlob Sentiment Lexicon
Contents
Update TextBlob Sentiment Lexicon#
Given the absence of several relevant descriptors from the textblob
sentiment lexicon, it is neccessary to update the lexicon with the missing terms.
The sentiment lexicon used by textblob
can be found at ...site-packages\textblob\en\en-sentiment.xml
The .xml file stores each word as an element with the following attributes:
form - the token itself
wordnet_id - WordNet3 lexical database ID number
pos - Penn Treebank POS tag
sense - indication of token usage
polarity - (negative/positive, -1.0 to +1.0)
subjectivity - (objective/subjective, +0.0 to +1.0)
confidence - specifies if an adjective was hand-tagged (1.0) or inferred (0.7)
For the purposes of this project, it is sufficient to populate only form and polarity.
Additionally, simply assigning polarity scores of 1.0 (positive) and -1.0 (negative) will also suffice.
Method#
1. Create dictionaries of relevant attributes#
iterate through missing positive and negative descriptors
create a dictionary for each descriptor containing relevant attributes for
sentiments.xml
.
import pandas as pd
negList=list(pd.read_csv("data/negList.csv")['Negative'])
posList=list(pd.read_csv("data/posList.csv")['Positive'])
df = pd.read_csv('data/tag_df.csv')
negWords = set(df[(df['sentiment']==0) & (df['description'].isin(negList))]['description'])
posWords = set(df[(df['sentiment']==0) & (df['description'].isin(posList))]['description'])
sen_dic = [{"form":word, "polarity":"1.0"} for word in posWords]
sen_dic.extend([{"form":word, "polarity":"-1.0"} for word in negWords])
2. Write new elements to sentiment.xml#
The ElementTree module (Python global module) is used to access and write to
sentiment.xml
Warning
It is advised to duplicate `sentiment.xml’ before performing any modifications
import xml.etree.ElementTree as ET
# Read original textblob sentiment xml file
tree = ET.parse("C:/Users/rob_t/anaconda3/envs/steam_nlp/Lib/site-packages/textblob/en/en-sentiment_org.xml")
root = tree.getroot()
# Iterate new sentiment dictionary and append new elements to xml
for x,y in enumerate(sen_dic):
elem = ET.Element("word",attrib=sen_dic[x])
elem.tail = "\n"
root.append(elem)
# write new snetiments.xml
tree.write("C:/Users/rob_t/anaconda3/envs/steam_nlp/Lib/site-packages/textblob/en/en-sentiment.xml")
3. Sentiment Scoring#
Sentiment polarity is re-assigned using updated sentiment lexicon
import numpy as np
# Overwrite df.sentiment with new scores
from textblob import TextBlob
df['sentiment'] = [TextBlob(x).sentiment.polarity for x in df['description']]
df['Sentiment1'] = np.select([(df['sentiment']>0),(df['sentiment']<0),(df['sentiment']==0)],['Positive','Negative','Neutral'])
EDA#
The sentiments lexicon is now updated.
Comparing the distribution of sentiment classifications before and after modifying the sentiments lexicon illustrates the appropriate frequency changes.
A reduction in the frequency of neutral descriptors and an associated increase in positive/negative descriptors is apparent.
import matplotlib.pyplot as plt
import seaborn as sns
sns.set_style('whitegrid')
fig, ax = plt.subplots(figsize=(9,7))
ax=sns.countplot(
data=pd.melt(df.loc[:,'Sentiment':]),
hue="value",
x="variable",
hue_order=['Negative','Positive','Neutral'],
palette=['#FF6F69','#88D8B0','#ffcc5c'],)
ax.set_xticklabels(['Before', 'After'], size=12)
ax.set_xlabel('Lexicon Modification', size=12)
ax.set_title("Compared Sentiment Distribution", size =14)
plt.legend(title='Sentiment', title_fontsize=12, loc='upper center')
plt.ylim(0,30000)
# create labels
for c in ax.containers:
labels = [f'\n\n {h/df.Sentiment.count()*100:0.1f}%' if (h := v.get_height()) > 0 else '' for v in c]
ax.bar_label(c, labels=labels, label_type='center')
ax.bar_label(ax.containers[0], label_type='center')
ax.bar_label(ax.containers[1], label_type='center')
ax.bar_label(ax.containers[2], label_type='center');
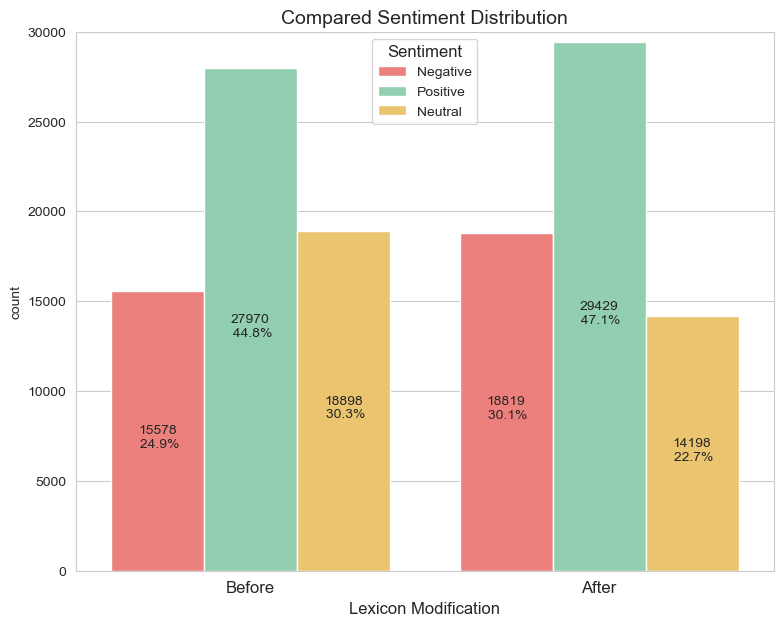